Jason Wang: Scientist Dedicated to Service
Senior Jason Wang successfully publishes a research paper on his novel machine-learning approach to cyberbullying detection and presents his findings at the IEEE BigData Conference.
Senior Jason Wang celebrates Lunar New Year and the snowfall that accompanies it.
February 16, 2021
Despite being notorious for limiting opportunities, the COVID-19 pandemic has simultaneously allowed room for ideas to prosper and projects to be pursued. For Jason Wang, a Jefferson senior, this meant carrying out research in cyberbullying detection and prevention through machine learning. As a student interested in not only computer science (CS) and artificial intelligence (AI), but also their social implications, he’s pursued opportunities to expand his experiences and skillset.
Since the summer of 2020, Wang has achieved success and recognition for his research, presenting his findings at the 2020 IEEE International Conference on Big Data in December and being awarded as one of just 300 semifinalists in The Regeneron Science Talent Search on Jan. 7—the nation’s oldest and most prestigious science and mathematics competition for high school seniors.
Amidst the COVID-19 pandemic, cyberbullying has become an even more serious threat. Our […] detection model is able to classify whether a cyberbully is targeting a victim’s age, ethnicity, gender, religion, or other quality. — Jason Wang, SOSNet: A Graph Convolutional Network Approach to Fine-Grained Cyberbullying Detection
Despite how far he has come in his journey, Wang traces his accomplishments back to his initial summer internship at Virginia Tech’s Discovery Analytics Center, where it all started.
“Basically in the spring, I reached out to different professors in the area, and I got an internship from one of them,” Wang said. “The entire purpose of the internship was to research, and we had the IEEE Big Data conference in mind. It’s sort of a way to measure your success in the internship.”
At the internship, Wang produced and published research paper, “SOSNet: A Graph Convolutional Network Approach to Fine-Grained Cyberbullying Detection,” advancing anti-cyberbullying measures and pushing towards a safer digital world.
“I would say that cyberbullying is a pretty important problem nowadays, especially since we have a lot more screen time,” Wang said. “The idea is that hopefully, big name social media companies will take this model and basically have an active cyberbullying detection feature. Because you know there’s the ‘Report’ button, but the problem is that nobody uses it: I’m pretty sure there’s a staggering statistic about how much goes unreported. So the idea is that you have the system that’s specifically rooting out the tweets, before any damage happens.”
However, conducting research wasn’t always a streamlined process for Wang. It took considerable trial-and-error with different models before reaching his most successful approach: using Graph Convolutional Networks (GCN).
“There were many approaches I tried that didn’t work as planned, but acted as stepping stones to my final GCN model,” Wang said. “The most valuable lessons I learned from my research experience were about perseverance and communication. In the face of these challenges, I had to speak up and be unafraid of sharing failures with my mentor, so that we wouldn’t get stuck in a single direction.”
Wang’s graph neural network algorithm can be used not only to detect cyberbullying, but also to categorize it.
“So the input is a tweet, and ideally you would have this scenario where each node in a graph is a tweet. Then similar tweets will have really strong connections, and then indistinct ideas will not really be connected at all,” Wang said. “The output is whether [the tweet] is cyberbullying—specifically, whether it’s a racist tweet or a sexist tweet, etc. And hopefully, that category will be right, and then we can flag that tweet saying, ‘Hey this tweet has problems, take it down.’”
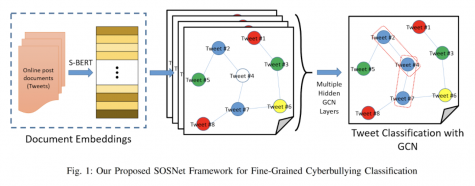
Throughout his process of research at his internship, Wang worked under the supervision of professor Chang-Tien Lu, who encouraged Wang to submit his research paper to the IEEE BigData conference, and Lu’s doctoral student Kaiqun Fu.
“I was working under one of [Dr. Lu’s] doctoral students, who were helping guide me through the research process,” Wang said. “So, we met on average twice a week for about an hour each, just to go through the entire research process from start to finish.”
Wang actively joined in on their weekly group seminar discussions, collaborating with senior students on advanced topics that bordered those of Wang’s research project. He values the teamwork and compounded ideas that played a crucial role in his project, expressing sincere gratitude towards anyone who helped him along the way.
“[The doctoral groups’ seminars I listened to] definitely helped guide my project—without them, I probably would not be here,” Wang said. “I feel very fortunate and I’m really grateful for all my CS teachers, mentors, and friends who supported me and helped cultivate my passion for machine learning.”
The IEEE BigData conference was held virtually this year, so the presentation of Wang’s research was pre-recorded for the audience. However, Wang answered questions live in the following Q&A segment, which he found to be a unique experience.
“The live question segment was definitely very nerve-wracking, because you’re surrounded by people who are much older and have a lot more experience with research,” Wang said. “But once [I got] into it, it wasn’t as terrifying as I thought. I think the questions were pretty enlightening, and it was nice to see that other people are taking an interest in my research.”
Reflecting on his experience and how it has impacted his future, Wang envisions himself conducting more research in CS in order to progress society forward.
“My greatest takeaway from this whole experience is that AI has a lot of power and potential to create positive impacts in our community,” Wang said. “This is just the start of my research career and in the future, I want to continue pursuing social change through AI research.”